The digital history of knowledge management and the advent of ChatGPT
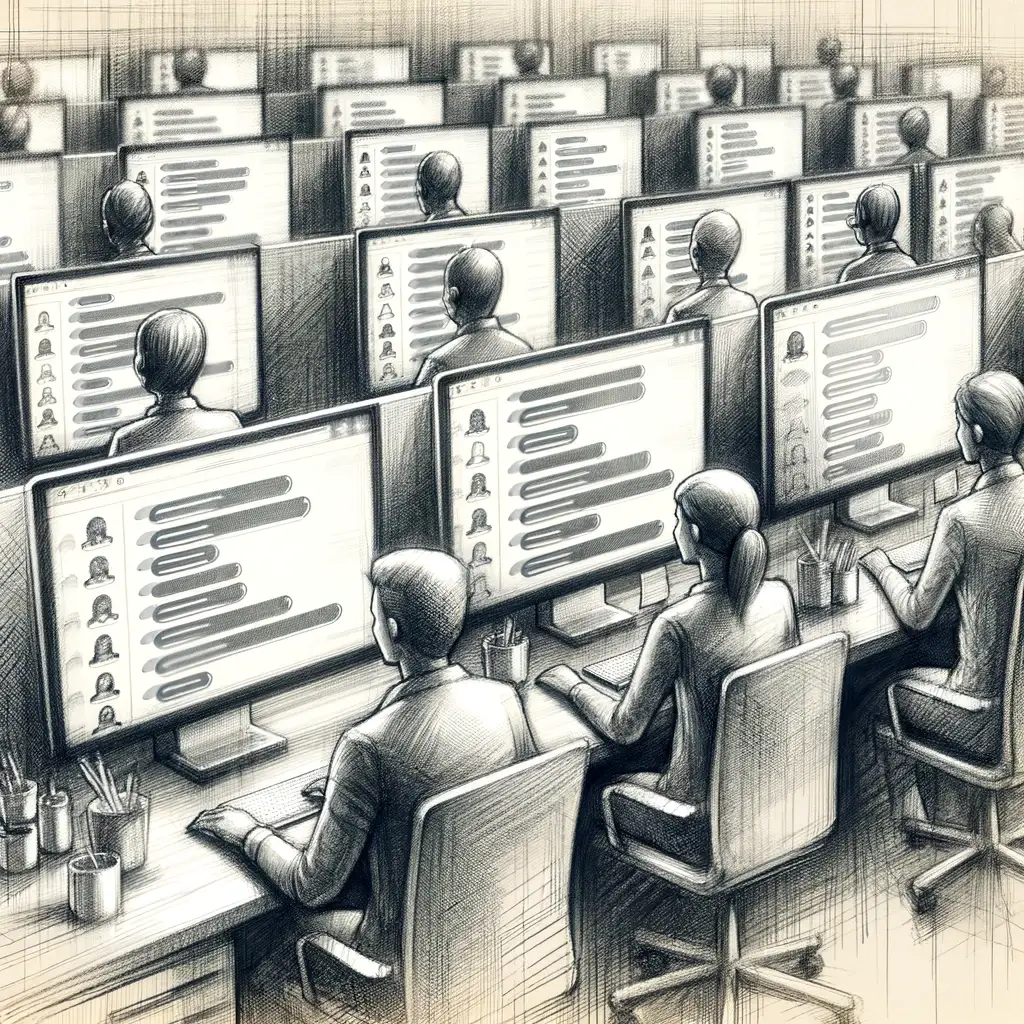
A year has passed since ChatGPT's launch, and amidst the buzz, it's clear that chatbot assistants have significantly impacted the knowledge work domain. Yet, the specifics of where and how they influence remain somewhat nebulous. To gain a clearer understanding, examining the effect of AI bots on knowledge management—a field that has seen modest progress since the advent of the read-write web, or social media—is essential. Given that content creation and sharing are crucial for knowledge management, what role does generative AI play in this process?
Over the past 15 years, I've observed a fascinating trajectory: a journey that began with the celebration of knowledge, transitioned into the data "revolution", and now, perhaps, is circling back to the core essence of knowledge itself.
To fully grasp the potential and implications of large language models like ChatGPT in the realm of knowledge management, considering the objectives of knowledge management and its digital evolution proves helpful.
The main three goals of knowledge management are:
- To find the right information where and when it's needed in the fastest possible way. This could be a document, video, audio file, or any type of codified information.
- To promote a culture of knowledge sharing and collaboration among individuals for better outcomes, recognizing that the majority of knowledge resides within people's minds, not merely in documents.
- To enhance and enrich the learning experience for individuals, aiming for the profound depths of wisdom. To borrow the words of David Weinberger, "The room is the smartest person in the room."
Why Large Language Models will be Critical for Knowledge Management
The significance of large language models (LLMs), which serve as the base for AI chatbots, becomes evident when examining the two main obstacles that have plagued knowledge management for the last 20 years: search and document management systems.
Search
Search engines have been essential for finding information on the ever-growing internet and have been mostly the starting point for research in the past 25 years. However, search engines have always been limited as they focus on a few keywords. Their results are heavily influenced by search engine optimization, and many search engines, particularly internal organizational ones, perform poorly to this day. Search engines deliver a lot of noise and little signal.
Large language models and their chatbot interfaces present a complete difference by focusing on the answer right from the start and hiding all the noise that led to the answer. This is a tremendous time-saver if the model has sufficient content from the requested knowledge domain and does not hallucinate in its replies. A language model that is thoroughly knowledgeable in its domain can yield satisfactory outcomes for numerous applications, potentially rendering many search engines obsolete in the imminent future.
Document Management System:
High hopes and large organizational investments went into document management systems that led to complex hierarchical document structures. These structures were not only time-consuming to navigate but, in many cases, did not add much value. A LLM that has analyzed all these documents and is able to answer questions based on them will replace these systems.
Organizational large language models will automatically analyze most documents residing on employees' laptops, depending on the data governance model, to provide better chatbot answers that automatically link to the respective source of information. Chat agents will learn from each person's specific work context and demands to provide better results.
Building Custom and Private Large Language Models for Better Information
Context is critical for large language models, and therefore custom or private GPT chatbots can provide much better results when they focus on the internal documents available in an organization. Thanks to open-source foundation models like LLaMA, it is now possible to customize them with one's own data, without the requirement to submit data to an external company. LLMs are very strong when it comes to ontologies, understanding how an organization's internal documentation is structured or logically building up its own information ecosystem (e.g. through fine-tuning).
These models have the ability to draw statistical conclusions based on connections within an organization, providing relevant information much more efficiently than older search algorithms. Think about how you navigate through folders and documents to find one specific piece of information, like finding a needle in a haystack.
Another level will be personal chatbots that for example OpenAI already offers, but this will be available to knowledge workers, where a personal chat assistant will have access to all personal documents, and you will be able to engage in a conversation with your own documents and content.
While internal and private GPT models have their benefits, they also come with risks. They are best suited for tasks that do not require precise information, such as improving the wording for a new brochure, as opposed to interpreting important guidelines. Even assessing the results and associated risks will require training, but it is challenging when these models are still considered a mystery, with even experts unable to fully understand how the bot generates each outcome.
Consequences for KM with Large Language Models
If we revisit the three main goals defined at the beginning, LLMs have the potential to enhance knowledge work, albeit only in some areas.
Finding Information: When ChatGPT systems improve their capabilities for niche subjects, they will provide valuable insights and resources for further exploration. LLMs excel in knowledge fields with abundant textual information available, suggesting stronger performance in areas with a high level of digital content and widespread discussions. However, they struggle with covering current events and topics with limited online presence. While codified knowledge is crucial for the functioning of LLMs, most of our understanding is implicit and cannot be easily translated into a coded form, highlighting their limitations.
Conducting a conversation with an Chatbot may decrease the initial search effort, but it does not necessarily save time when it comes to thoroughly understanding the content. In some cases relying on chatbots for summarization can potentially prevent you from encountering crucial information that may be relevant.
Another challenge remains that every foundational language model inherently contains certain biases because they are created from what information is available on the Internet and that is not neutral. For instance, there is a significant bias towards English content compared to other languages. Codified knowledge can only provide one perspective of viewing the world.
In Knowledge Sharing:
They fall short in this aspect; this is the critical level of implicit knowledge, where our experiences and non-codified knowledge are essential. Imagine a chatbot attempting to teach you how to ride a bicycle. It may offer some assistance, but it can never fully teach you. In complex situations where knowledge exchange is necessary, LLMs do not hold much value as they are limited to only codified information, which is a small fraction of human knowledge. Interestingly, AI models are already facing challenges due to lack of available data at this point.
Learning:
When it comes to learning, differentiating between implicit (experiential) and tacit (codified) knowledge is important. For instance, chatbots are not very useful for imparting implicit knowledge, as demonstrated by the example of riding a bicycle. Navigating complex intercultural conversations requires experiential knowledge that can only be gained through practice. On the other hand, chatbots can serve as great question and answer partners for transferring tacit knowledge, such as staying up-to-date with guidelines or learning about new topics related to one's work domain. This is because the best way to learn is by testing your own knowledge and integrating it with your existing understanding.